Effective risk management is the key to the success of an AI prediction of stock prices. If managed properly, these factors can reduce possible losses while maximizing returns. Here are ten tips to consider these factors.
1. Assessment of the Stop-Loss Levels and Take Profit Levels
What are the reasons: These levels reduce the risk of losses and secure profits by limiting exposure to extreme market fluctuations.
How: Check that the model is dynamic and has rules for take-profit, stop-loss and risk factors that are based on the volatility of the market or other risk factors. Models with adaptive parameters perform better in a variety of market conditions. They also aid in prevent excessive drawdowns.
2. Assess the risk-to-reward ratio considerations
The reason: A high ratio of risk to reward assures that profits will outweigh risks, and also ensures sustainable returns.
How: Verify that the model is able to define an appropriate ratio of risk to reward per trade. For instance 1:2 or 1:
3. This is an excellent indicator of the possibility that models will make better decisions and limit high-risk trading.
3. Make sure you are aware of the maximum drawing down Constraints
What's the reason? Limiting drawdowns of the model will stop it from suffering huge losses that are difficult to recuperate.
How to: Make sure that the model includes drawdown limits (e.g. 10 percent). This constraint is a great way to lower long-term risk and protect capital during downturns in the market.
Review strategies to size positions dependent on risk to the portfolio
Why: Position size determines how much capital will be allotted to each trade. It balances returns and risk.
How do you determine whether the model employs the risk-based approach to sizing that means that the size of the position trade is adjusted according to the risk of the investment, the individual risk associated with trading, or the overall risk to the portfolio. The result of adaptive positioning is more balanced and less vulnerable portfolios.
5. Look for Position Sizing that is Volatility Adjusted
Why: Volatility -adjusted size means that you can take larger positions in assets that are less volatile while taking smaller ones on high-volatility investments, thus improving your stability.
Verify that the model employs an adjusted volatility scale, such as the average true range (ATR) of standard deviation. This will help to ensure that the risk exposure is consistent across every trade.
6. Confirm Diversification across Sectors and Asset Class
Diversification can reduce the risk of concentration through the spread of the investments across different types of assets or industries.
How: Check to see whether the model has been programmed for diversification, especially when markets are volatile. A model that is well-diversified will help reduce losses when a particular sector is declining and help keep the portfolio in a stable state.
7. Evaluate the benefits of using Dynamic Hedging Strategies
Why? Hedging limits exposure to potential adverse market movements and protects capital.
How: Verify whether the model is using methods of hedging dynamically like options or inverse ETFs. Hedging effectively can help stabilize performance in volatile markets.
8. Assess Adaptive risk Limits Based on Market Condition
Reason: Because markets are not the same It isn't a good idea to establish fixed risk limits for all scenarios.
How do you verify whether the model is altering risk limits in line with volatility or sentiment in the market. Flexible risk limits enable models to take on more risk when markets are stable and reduce exposure in times of uncertainty.
9. Verify the Realtime Monitoring Portfolio Risk
Why: Real-time risk monitoring lets the model respond immediately to market changes and minimize the chance of losing.
How: Look for tools that monitor real-time portfolio metrics like Value at Risk (VaR) or drawdown percentages. A model that has live monitoring will be capable of reacting to market changes that are sudden and minimize the risk you take.
Review Stress Testing and Scenario Analysis of Extreme Events
Why is this? Stress testing can aid in predicting the performance of a model in difficult conditions like financial crises.
What to do: Make sure that the model has been stress-tested against historical financial and market crises. This will help assess its durability. Scenario analysis helps make sure that the model is able to stand up to sudden declines without significant losses.
You can assess the robustness and efficiency of an AI model by following these tips. A well balanced model can be able to balance rewards and risk to ensure consistently good results under different market conditions. Read the top rated ai for stock trading examples for website recommendations including ai ticker, ai stock price prediction, stock technical analysis, best ai trading app, investing in a stock, stock technical analysis, stock market how to invest, predict stock market, ai technology stocks, ai stock prediction and more.

Top 10 Tips For Assessing The Nasdaq Composite By Using An Ai-Powered Prediction Of Stock Prices
Assessing the Nasdaq Composite Index using an AI stock trading predictor requires knowing its distinctive characteristic features, the technology-focused nature of its components, and how well the AI model is able to analyze and predict its movement. Here are 10 suggestions to help you evaluate the Nasdaq composite with an AI stock trading prediction:
1. Learn more about the Index Composition
Why? The Nasdaq composite comprises more than 3000 companies, mainly in the technology, biotechnology and internet industries. This sets it apart from a more broad index such as the DJIA.
How do you: Be familiar with the largest and influential companies in the index, including Apple, Microsoft, and Amazon. Knowing their significance can help AI better predict movement.
2. Incorporate specific elements for the sector.
Why? Nasdaq is largely influenced by technology trends and specific events in the sector.
How can you make sure that the AI model is based on relevant variables like tech sector performance, earnings reports, as well as trends in the software and hardware industries. Sector analysis improves the predictive power of an AI model.
3. Utilize the Technical Analysis Tool
The reason: Technical indicators aid in capturing market sentiment and also the trend of price movements in an index that is as unpredictable as the Nasdaq.
How do you use techniques of technical analysis like Bollinger bands or MACD to integrate into your AI model. These indicators will help you spot buy and sale signals.
4. Monitor the Economic Indicators That Impact Tech Stocks
Why: Economic variables like interest rates as well as inflation and unemployment rates are able to significantly affect tech stocks, the Nasdaq, and other markets.
How to integrate macroeconomic factors that affect the technology industry including technology investment, consumer spending trends as well as Federal Reserve policies. Understanding these relationships improves the model's accuracy.
5. Earnings report have an impact on the economy
The reason: Earnings announcements by large Nasdaq companies could trigger substantial price fluctuations and impact the performance of the index.
How: Ensure the model tracks earnings calendars and adjusts predictions based on the dates of earnings releases. It is also possible to increase the accuracy of predictions by analyzing the reaction of historical prices to earnings announcements.
6. Use Sentiment Analysis for tech stocks
Why? Investor sentiment can have a significant impact on the value of stock, especially in the tech industry in which trends can rapidly change.
How do you integrate sentiment analysis of financial news as well as social media and analyst ratings in the AI model. Sentiment metrics is a great way to provide additional context and enhance predictive capabilities.
7. Backtesting High Frequency Data
Why? The Nasdaq has a reputation for high volatility. It is therefore crucial to verify predictions using high-frequency data.
How to use high-frequency data to test the AI model's predictions. It helps validate its performance across a range of market conditions.
8. Examine the model's performance under market adjustments
The reason is that Nasdaq's performance could be drastically affected during downturns.
What to do: Study the model's past performance, particularly in times of market declines. Stress testing can reveal the model's resilience and its ability of mitigating losses during volatile times.
9. Examine Real-Time Execution Metrics
Why? Efficient execution of trades is vital for capturing profit, especially with a volatile index.
What are the best ways to track the execution metrics in real-time including slippage and fill rates. Test how accurately the model can predict optimal times to enter and exit for Nasdaq related trades. This will ensure that the execution corresponds to predictions.
10. Validation of Review Models using Ex-Sample Testing Sample Testing
Why? Testing out-of-sample helps to ensure that the model can be generalized to the latest data.
How can you use historic Nasdaq trading data that is not used for training to conduct rigorous tests. Examine the model's predicted performance against actual results to ensure the accuracy and reliability.
You can evaluate the capabilities of an AI trading predictor to reliably and accurately analyse and forecast Nasdaq Composite Index movements by following these tips. Check out the top stock market ai blog for website examples including ai companies stock, stock pick, stock investment prediction, ai share trading, ai stock investing, ai stocks to buy now, predict stock market, top ai companies to invest in, ai to invest in, open ai stock symbol and more.
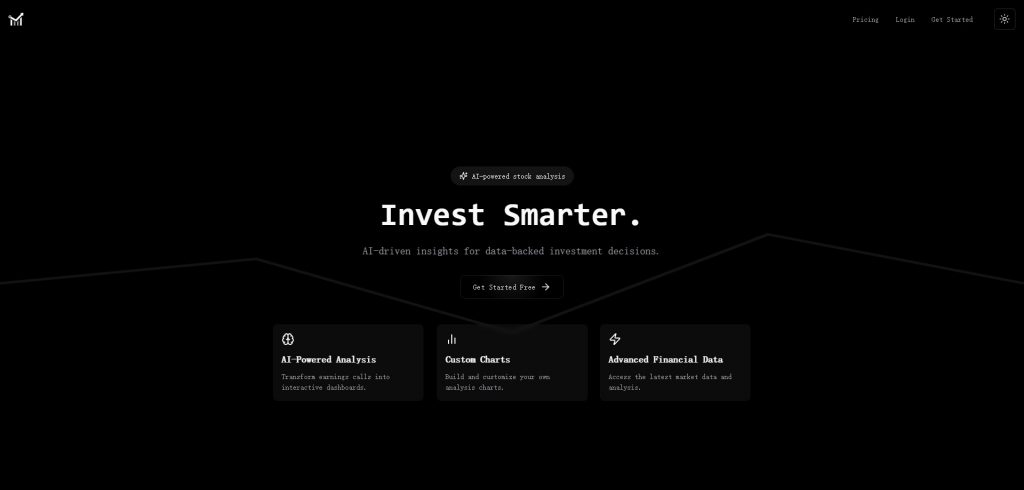